Correlative Microstructure Analysis and Release Prediction for a Parenteral Implant

Correlative Image-Based Release Prediction and 3D Microstructure Characterization for a Long Acting Parenteral Implant
Imaging-based characterization of polymeric drug-eluting implants can be challenging due to the microstructural complexity and scale of dispersed drug domains and polymer matrix. The typical evaluation via real-time (and accelerated in vitro experiments not only can be very labor intensive since implants are designed to last for 3 months or longer, but also fails to elucidate the impact of the internal microstructure on the implant release rate. A novel characterization technique, combining multi-scale high resolution three-dimensional imaging, was developed for a mechanistic understanding of the impact of formulation and manufacturing process on the implant microstructure. Artificial intelligence-based image segmentation and imaging analytics convert “visualized” structural properties into numerical models, which can be used to calculate key parameters governing drug transport in the polymer matrix, such as effective permeability. Simulations of drug transport in structures constructed on the basis of image analytics can be used to predict the release rates for the drug-eluting implant without running lengthy experiments. Multi-scale imaging approach and image-based characterization generate a large amount of quantitative structural information that are difficult to obtain experimentally. The direct-imaging based analytics and simulation is a powerful tool and has potential to advance fundamental understanding of drug release mechanism and the development of robust drug-eluting implants.
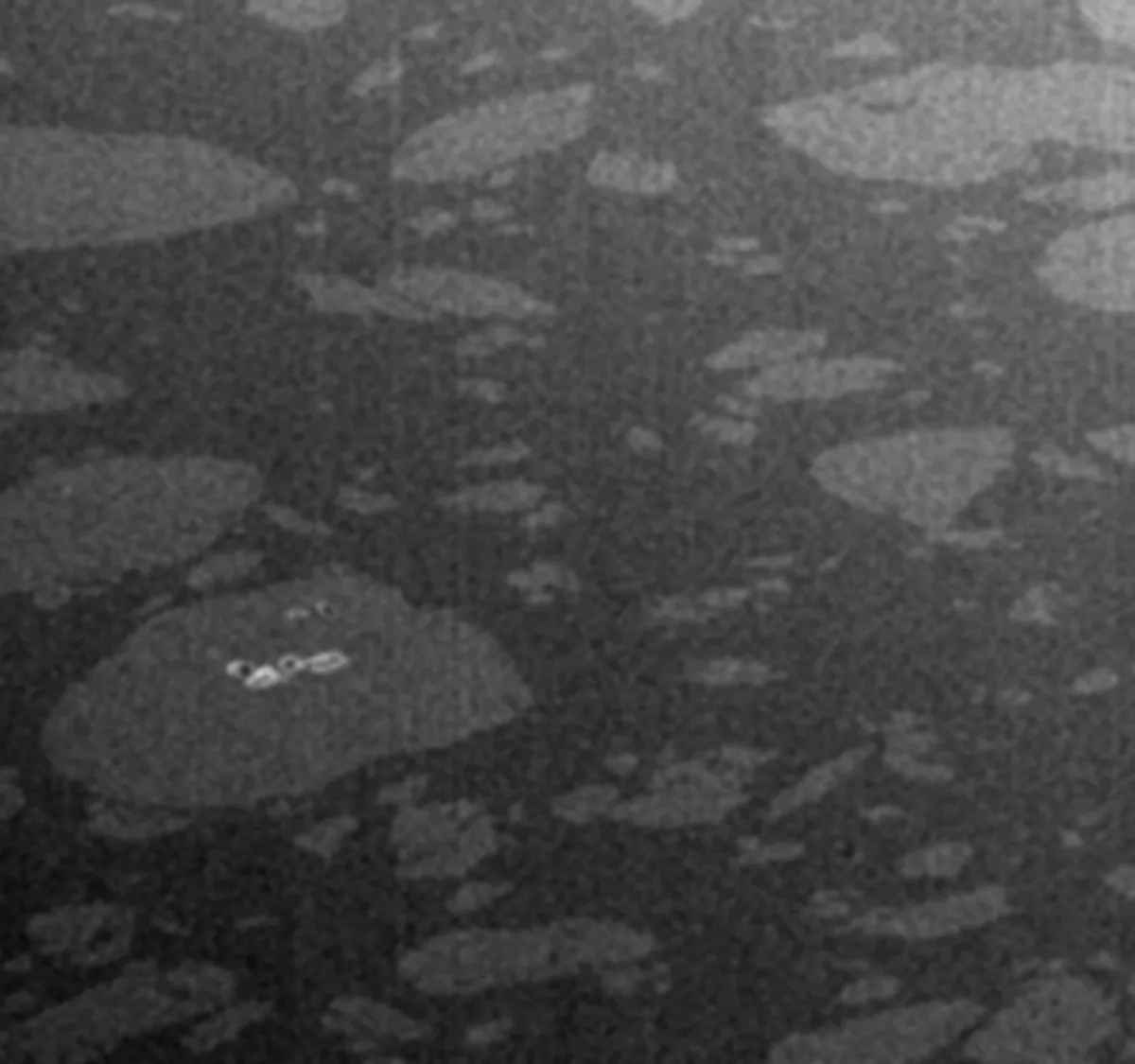
Zhen Liu, Li Li, Shawn Zhang, Joshua Lomeo, Aiden Zhu, Jacie Chen, Stephanie Barrett, Athanas Koynov, Seth Forster, Peter Wuelfing, Wei Xu
Published with Merck
https://doi.org/10.1007/s11095-021-03145-2
Additional Publications
Transform Your Program with Microstructure Science
Get started with a drug product digital twin.
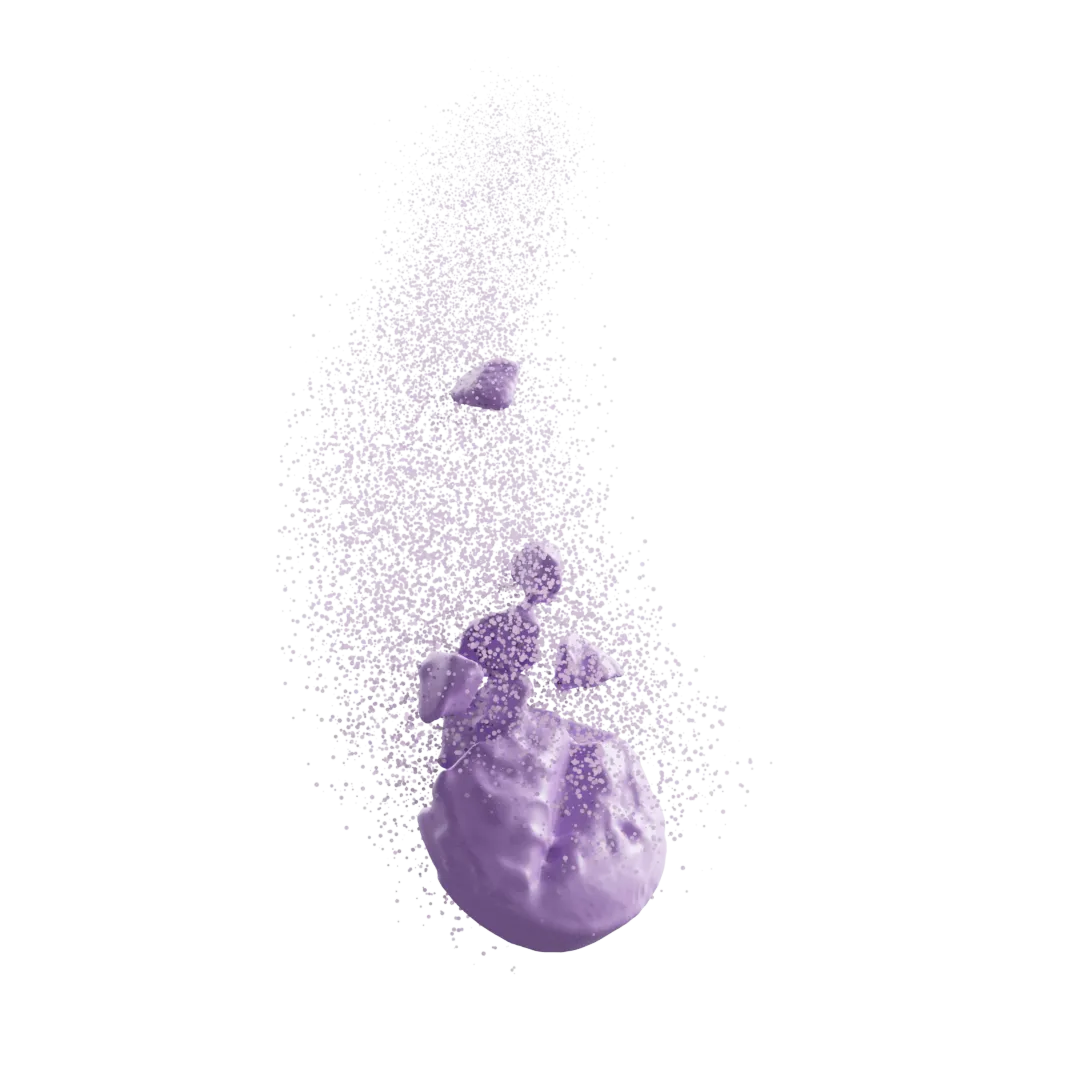